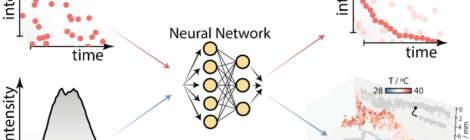
Two exciting talks from Universidad Autonoma de Madrid in our seminar on March 6
Exploration of catalytic thickness effect for enhanced hydriding kinetics in magnesium thin films
Paula Prieto Porras1, Fabrice Leardini1, Isabel J. Ferrer1, Carlos Morales2 and Jose Ramón Ares1
1 MIRE group, Dpto. Física de Materiales, Universidad Autónoma de Madrid, Madrid, España
2 Applied Physics and Semiconductor Spectroscopy, Institute of Physics, Faculty 01, Brandenburg University of Technology Cottbus-Senftenberg. Germany
Magnesium (Mg) has emerged as a promising candidate for optical hydrogen sensing, switching between reflective and transparent states upon hydrogenation as it undergoes a strong metal-to-insulator transition [1]. Despite the potential of the material, thermodynamic and kinetic restrictions, as well as its high reactivity, hinder its practical use [2,3].
To overcome these difficulties, Mg-films are usually coated with an ultrathin layer of palladium (Pd) to prevent the formation of oxides/hydroxides onto the Mg-surface and reduce the hydrogen dissociation barrier [4]. Although this catalytic layer significantly reduces the hydrogenation temperature (from 300 ºC to 100 ºC), the explanation of the palladium’s influence on H-absorption kinetics is still debated due to the variety of phenomenology involved at the film surface/interface [5], such as Mg-Pd interdiffusion or phase nucleation among other possible variables. In this work, the influence of the catalytic palladium thickness (from 1 to 30 nm) on the H2 absorption near ambient temperatures (< 80°C) has been investigated. For this purpose, Pd-capped Mg films were deposited using e-beam evaporation and structurally and morphologically characterized using X-ray diffraction (XRD), profilometry, and field emission gun scanning electron microscopy (FEG-SEM), respectively.
To unveil the underlying absorption mechanisms, “in situ” optical transmittance measurements during H2 absorption process (P(H2 )= 1 bar at T = 45, 60, and 80 ºC) were performed. Obtained results show (i) a drastic influence of Pd- thickness on H-kinetics and (ii) a two-step control mechanism (nucleation and growth and H-diffusion) in the hydrogenation process. To discern the underlaying causes that alter the kinetics of the absorption reaction as a function of the Pd thickness, a complete set of characterization techniques were used, providing an accurate morphological, structural and compositional characterization of the film’s surface and interface before and after the hydrogenation process (Fig.1).
Machine learning in luminescence nanothermometry
Liyan Ming, Erving Ximendes, Riccardo Marin, Daniel Jaque
Nanomaterials for Bioimaging Group (nanoBIG), Departamento de Física de Materiales, Facultad de Ciencias, Universidad Autónoma de Madrid, Madrid, Spain,
Email: liyan.ming@estudiante.uam.es
Luminescent nanothermometry utilizes changes in nanoparticle luminescence properties to provide remote, minimally invasive temperature readouts in biological systems, including thermal therapies, brain monitoring, and inflammation detection. However, challenges remain in achieving precise and reliable measurements, particularly under low signal-to-noise ratio (SNR) conditions.[1-2] Machine learning algorithms, particularly convolutional neural networks (CNNs), offer promising solutions to improve spatiotemporal resolution and reliability. For instance, a U-Net model was applied to enhance thermal readout precision by converting luminescence decay curves into images, enabling the algorithm to learn relevant features from noisy data and improve temperature estimation. Furthermore, while current techniques provide only average or 2D thermal images, accurate 3D thermal maps are crucial for biological applications. Our recent approach, using Ag2S nanothermometers and CNNs, overcomes this limitation by extracting 3D thermal maps from luminescence data, leveraging distortions in the emission spectra caused by temperature and tissue-induced photon extinction. This optimized method provides precise 3D thermal maps of complex nanothermometer patterns.
Figure. Machine learning enabled reliable luminescence thermometry (LT) in extreme low signal to noise ratio (SNR) conditions, and 3D thermal imaging.
References
[1] C.D. Brites, R. Marin, M. Suta, et.al, Adv. Mater., 35(36), 2302749.
[2] A. Bednarkiewicz, M. Lukasz, L. D. Carlos, et.al, Nanoscale 2020, 12, 14405-14421.
The seminar takes place at 15:00 Berlin time (this is 14:00 in Lithuania)
For the link to the seminar check the poster or contact Katharina Rubahn.